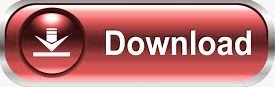
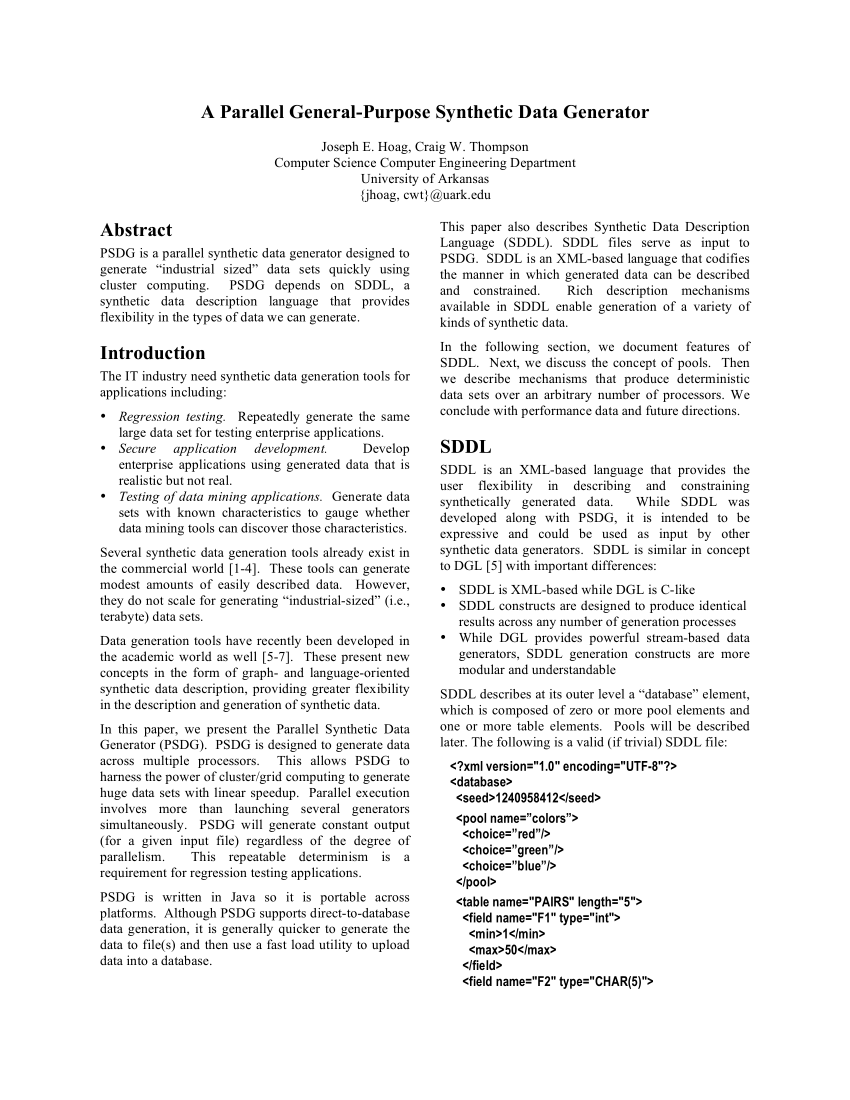
The creation of machine learning prediction models can add more value to tabular data.ĭeep learning models’ synthetic data has demonstrated success in predictive modeling tasks. They can assist in facilitating researchers’ access to private information, bridging data availability gaps for agent-based simulations, artificial control approaches, and counterfactual research. To solve data privacy concerns and sparsity, synthetic tabular data might be helpful. While generative models for text and graphics are ubiquitous, there are few models for creating artificial tabular data, despite the wide range of potential uses. 🎟 Be the first to know the latest AI research breakthroughs. Synthetic picture-generating models like DALLE and, most recently, ChatGPT have made generative models widely used. In recent years, there has been substantial advancement in creating synthetic data. This could be because simulating the intricate interactions between and within tables takes a lot of work. Despite its widespread use, only a small amount of effort has been made to creating artificial relational datasets. A parent table is non-relational tabular data in the context of a relational dataset, whereas the child table is relational tabular data. Relational tabular databases simulate logical data partitioning and avoid duplicating observations between parent and child tables. A relational dataset consists of at least one pair of tabular data files connected by a single identifier and containing a one-to-many mapping of observations between the parent table and the child table, respectively. Relational tabular data are tabular data that have observations that are connected. Non-relational tabular data are tabular data that contain observations that are unrelated to one another. o i =, where j denotes the j th column, defines a single observation in a data table with n columns. Tabular data is formally a group of observations (rows) o i that may or may not be independent. The generative models used to create these synthetic data must ensure that “data copying” won’t take place to comply with data privacy regulations. A better option is synthetic tabular data, which adds greater value, particularly in granular and segmentation analysis, and has statistical features similar to real data. Differential privacy techniques, homomorphic encryption strategies, or federated machine learning may be used to implement, enabling researchers access to insights from sensitive data. Even after using statistical disclosure methods, they might still be the target of hostile assaults because their low utility results from their constrained dispersion.

These datasets could include private information that shouldn’t be made public. This form contains many datasets from surveys, censuses, and administrative sources. The most prevalent type of data is tabular data.
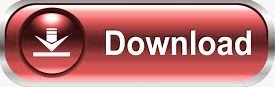